Deep physical neural networks trained with in-situ backpropagation
Seminar
- Datum: 08.09.2023
- Uhrzeit: 10:30 - 12:00
- Vortragender: Dr. Tatsuhiro Onodera
- NTT Research’s PHI Laboratory, Sunnyvale, CA, and Cornell University, School of Applied and Engineering Physics, Ithaca, NY
- Ort: Max-Planck-Institut für Mikrostrukturphysik, Weinberg 2, 06120 Halle (Saale)
- Raum: Lecture Hall, B.1.11
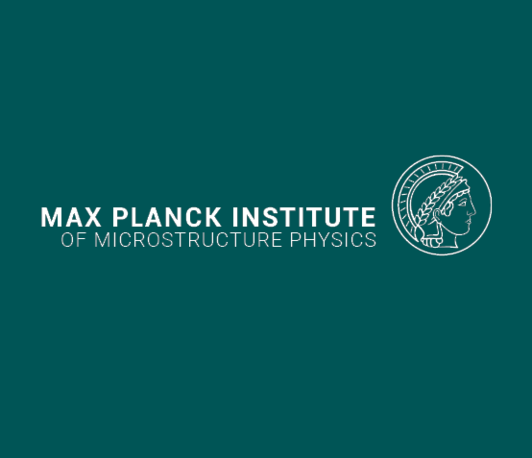
Deep learning excels in complex tasks like natural language processing and machine vision due to its ability to learn hierarchical representations from data. However, escalating energy consumption increasingly limits its scalability, which has motivated the development of specialized hardware for energy-efficient deep learning. Traditionally, this revolved around computing matrix-vector computations, but this approach limits the array of physical systems that can be deployed to solve deep learning's energy problem.
The talk will center
around our recent work on deep physical neural networks, which leverage
controllable physical systems for deep learning. These networks utilize
the inherent transformations of their physical
components to effectively learn hierarchical representations of input
data, akin to traditional deep neural networks. For efficient training,
we introduce a novel hybrid in situ - in silico backpropagation
algorithm. We demonstrate the generality of our proposed
framework by experimentally constructing physical neural networks from
multimode mechanical oscillators, analog electronic circuits, and
ultrafast nonlinear optical systems, to perform image classification
tasks. Beyond these proof-of-concept experiments,
we have also probed the potential of scaled-up physical neural networks
through simulations. Finally, I will discuss our recent work on
constructing a physical neural network with a large number of parameters
that is based on integrated photonics.