Programmable photonics for deep neural network inference and training
Seminar
- Datum: 08.09.2023
- Uhrzeit: 14:00 - 15:00
- Vortragender: Dr. Saumil Bandyopadhyay
- Massachussetts Institute of Technology, Quantum Photonics Laboratory
- Ort: Max-Planck-Institut für Mikrostrukturphysik, Weinberg 2, 06120 Halle (Saale)
- Raum: Lecture Hall, B.1.11
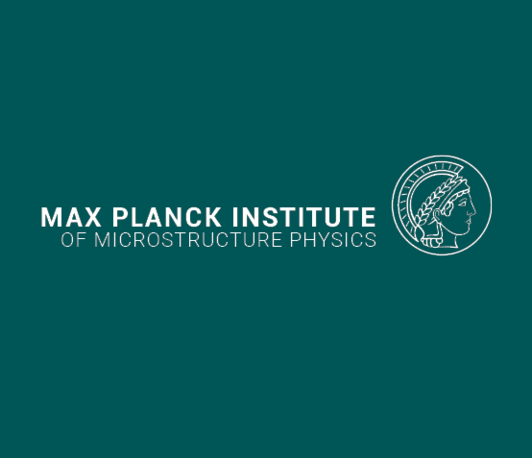
Exponential scaling
of the size of deep neural networks (DNNs) has motivated
the development of new hardware architectures optimized
for artificial intelligence models. At the same time,
advances
in the fabrication of large-scale integrated silicon photonics have sparked
interest in optical systems as a platform for processing DNNs at high speeds with
ultra-low energy consumption.
Although mapping linear
algebra to photonic hardware is relatively straightforward, implementing a fully-integrated photonic platform for DNN processing, which performs both linear and nonlinear computation on a single chip, has remained an outstanding
challenge.
In this talk, I will discuss our recent work towards realizing such a system in silicon photonics.
I will first discuss the development of error correction algorithms for programmable photonic processors, whose capabilities are believed to be limited by fabrication error. By applying deterministic, gate-by-gate error correction, we show that these systems, despite being constructed from imprecise, analog components, can be efficiently programmed to implement highly accurate linear matrix processing suitable for machine learning models.
I will then discuss the development and demonstration of a single-chip, end-to-end silicon photonic processor for DNNs. This fully integrated coherent optical neural network, which monolithically integrates multiple photonic processor units for matrix algebra and nonlinear activation functions into a single silicon chip, eliminates optical-to-electrical conversions between layers and implements single-shot coherent optical processing of a DNN with sub-nanosecond latency. We demonstrate that this system can directly train DNNs in situ, obtaining high accuracies on a vowel classification task comparable to that of a digital system. Our results open the path towards integrated, large-scale optical accelerators for low-latency DNN inference and training.