Successful PhD defense of Kai-Uwe Demasius
Congratulations to Kai-Uwe Demasius!
Title: "Highly energy efficient neuromorphic computing based on memcapacitive devices" |
Abstract
Data transfer between memory and the processor in digital von Neumann architectures consumes a large amount of energy. This becomes extremely critical in modern data-intensive tasks, such as neural network training. Recently, neural networks were mapped onto arrays of resistive non volatile memory for highly parallel multiply-accumulate operations. (Mem)-capacitive devices can similarly be employed with the advantage of lower static power consumption, but they suffer from a poor dynamic range and scalability. In this thesis a CMOS-compatible (mem)-capacitive device based on charge screening is proposed, theoretically simulated and experimentally demonstrated. Scalability down to 45nm-90nm is shown by simulations, while retaining a large capacitance dynamic range. By using concepts of adiabatic charging it is shown that mapping neural network inference tasks with 6-8 Bit precision can be done with 30-300x better energy efficiency compared to common state-of-the-art resistive technologies and possibly greater efficiency than the human brain. Experimental devices and crossbars were fabricated at the micrometer scale and an image recognition algorithm with letters “M”, “P” and “I” is shown on 156 synaptic devices.
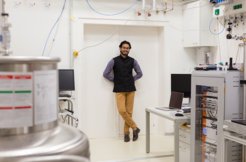