Ultra-low-power memcapacitor device for neuromorphic computing
Much effort is being made to develop new types of memory devices that can revolutionize the computing paradigm of the past half century. Major breakthroughs are needed, in particular, in the domain of Deep Learning as a subset of Artificial Intelligence. In-memory computing and brain inspired neuromorphic hardware are the driving principles for this paradigm shift. Recently, a team of scientists at the Max Planck Institute of Microstructure Physics, Halle (Saale), and SEMRON GmbH, Dresden, Germany, have successfully demonstrated a unique capacitive memory technology with unprecedented energy-efficiency. The technology can easily be implemented using conventional silicon manufacturing processes and systems, thereby paving the way to its rapid commercialization and adoption in many application domains. The results were published in Nature Electronics on October 11th.
Mainstream computing architectures used since the 1970s are based on a von Neumann architecture with distinct memories and processors. With the rise of Deep Learning (DL), a new class of ultra-parallel algorithms has emerged. The current architectures employed in CPUs and GPUs consume much energy in transferring data between memories and processors. This problem can be addressed by “in-memory computing” where the multiplication and accumulation operation (MAC), which is the fundamental operation in most commercial DL applications today, is carried out within the memory itself. One common approach is to use analog resistive memory devices, such as memristors or phase-change memory, where the multiplication is carried out by Ohm´s law and the accumulation by Kirchhoff´s current law. Capacitive devices are inherently superior to resistive devices with regard to power consumption due to their higher signal-to-noise ratio. Kai-Uwe Demasius, CTO of SEMRON and co-author of the paper says: “All previous capacitive approaches suffer either from impractical fabrication or from low precision”.
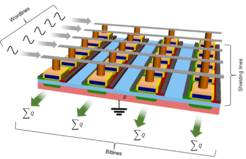
These problems are solved in this research work by making use of a charge shielding layer, which can either transmit or strongly shield an electric field. The shielding layer’s characteristics can be controlled either by applying an external voltage to a doped junction or by the influence of a non-volatile memory in an upper dielectric layer. There are many possible implementations of this novel memory cell. While commercialization favors approaches without any new materials, a ferroelectric material was used in this work since it allows for ultra-low power characteristics in writing the DL model parameters. The capacitive memory devices are arranged in a crossbar array to enable highly parallel vector-matrix multiplications.
The researchers fabricated a prototype crossbar array with 156 memory cells and successfully implemented a 5x5 image recognition algorithm for classification of the letters M, P and I. A high dynamic range of 1:1478 and remarkable analog programming capabilities were shown for single devices. Moreover, detailed simulations showed a good match between the experimental and simulated results, with scalability demonstrated for devices as small as 45 nm in size. Ultra-high energy efficiency was established using the concept of adiabatic charging to thereby realize an energy efficiency that exceeds 3,500 TOPS/W (Tera operations per second per Watt) with 6-8 bit precision. For a handwritten digit algorithm an energy efficiency of 29,600 TOPS/W was obtained, that is the highest energy efficiency ever reported for a neuromorphic system at this precision. “This efficiency far exceeds that of the human brain, which is assumed to be in the range of 100 TOPS/W”, according to the director of the Max Planck Institute of Microstructure Physics, Stuart Parkin.
The publication entitled “Energy-efficient memcapacitor devices for neuromorphic computing” was published on October 11th in the journal Nature Electronics.
DOI: 10.1038/s41928-021-00649-y
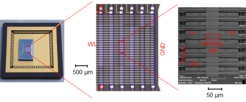